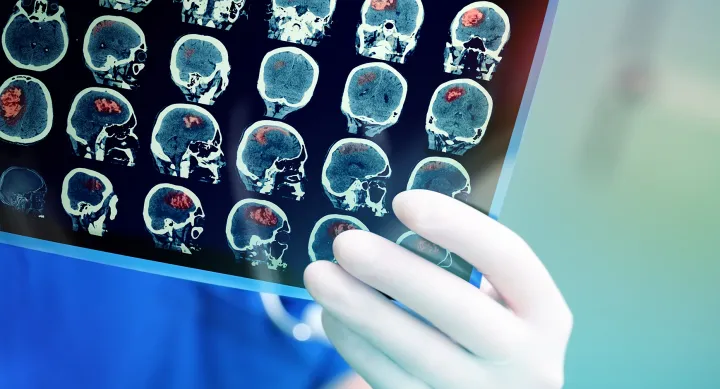
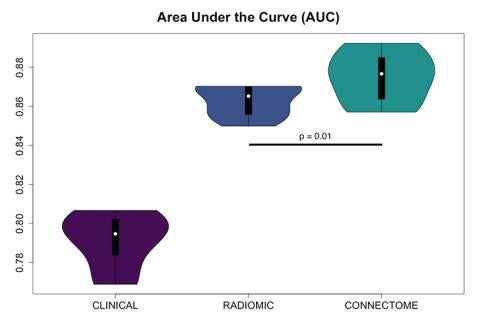
Diffuse gliomas are the most common malignant primary brain tumor. Histologic grade along with several other demographic, disease, and treatment-related variables are associated with prognosis, but wide variation in patient outcome remains. Predicting survival from baseline characteristics is especially useful in risk stratification and planning individualized therapy. Brain magnetic resonance imaging (MRI) is frequently used for surgical planning and is therefore available for most glioma patients. Neuroimaging provides unique advantages as a biomarker for neurologic conditions given that the brain reflects biologic, molecular and environmental processes. Previous studies have attempted to predict glioma outcome using tumor-based imaging features, but these methods have ignored the predictive capacity of the entire brain network.
Our preliminary studies show that features extracted from the entire brain network outperform tumor-limited features in predicting overall survival in patients with glioma. The purpose of our GLIOMA study, funded by the National Cancer Institute, is to validate the performance of our preliminary machine learning prediction models in a larger sample (N = 1,500). We will also examine brain network organization alterations associated with O(6)-methylguanine-DNA methyltransferase (MGMT) promoter methylation.
Contact Information
Director: Shelli Kesler, PhD
Please contact us to discuss how you can participate by emailing at kesler.lab@austin.utexas.edu.